A machine-learning approach for detection of mobile replicons in metagenomics
Doctoral Researcher:
M.Sc. Clara Emery, GEOMAR - Helmholtz Centre for Ocean Research Kiel, cemery@geomar.de
Supervisors:
- Prof. Dr. Ute Hentschel Humeida, GEOMAR - Helmholtz Centre for Ocean Research Kiel, Marine Ecology, uhentschel@geomar.de
- Prof. Dr. Tal Dagan, University of Kiel, Institute for Microbiology, tdagan@ifam.uni-kiel.de
Location: Kiel
Disciplines: Bioinformatics, Machine-Learning, Marine Microbiology
Key words: Metagenomes, Mobile replicons, Machine-learning, Marine sponges
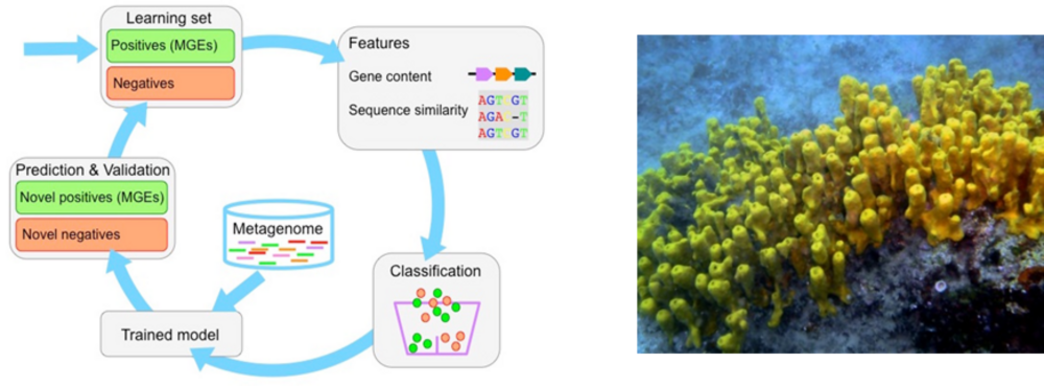
Background: The study of microbial species diversity and function using metagenomics – i.e., the direct sequencing of DNA from the environment – has become a standard practice in environmental microbiology. The application of metagenomics is especially useful for the study of microbial communities including strains that cannot be cultivated in laboratory conditions. Nonetheless, a long-standing challenge in the analysis of metagenomics is the classification of the resulting sequences according to their replicon type and taxonomic origin. Mobile replicons – including bacteriophages and plasmids – are of special interest for the study of microbial communities as they may encode for functions that are laterally transferred within, or into, the community.
Aim: The overarching goal is provide novel information on the nature and function of different mobile elements which may have important implications for a microbial lifestyle within animal hosts.
Objectives: The proposed PhD project aims to develop a computational toolbox for the detection of mobile replicons with a focus on plasmids and bacteriophages in metagenomics data. The specific tasks are as follows: (i) develop a machine-learning approach for the detection of mobile replicons according to gene content and order. (ii) identify and optimize the optimal feature set as well as test of various machine-learning algorithms. (iii) use existing metagenomics data from marine sponge-associated communities, which contain a diverse repertoire of mobile genetic elements including conjugative plasmids, transposons, integrons and phages.
References:
- Burstein D, Gould SB, Zimorski V, Kloesges T, Kiosse F, Major P, Martin WF, Pupko T, Dagan T (2012) A machine learning approach to identify hydrogenosomal proteins in Trichomonas vaginalis. Euk Cell 11:217–228. doi: 10.1128/EC.05225-11
- Moitinho-Silva L, Steinert G, Nielsen S, Hardoim CCP, Wu YC, McCormack GP, López-Legentil S, Marchant R, Webster N, Thomas T, Hentschel U (2017) Predicting the HMA-LMA status in marine sponges by machine learning. Front Microbiol: doi: 10.3389/fmicb.2017.00752
- Slaby BM, Hackl T, Horn H, Bayer K, Hentschel U (2017) Metagenomic binning of a marine sponge microbiome reveals unity in defense but metabolic specialization. ISME J 11(11): 2465-2478. doi: 10.1038/ismej.2017.101.