Cross-Domain Marine Data Fusion: Discover Knowledge on Open Ocean Oyxgen Minimum Zones.
Doctoral Researcher:
M.Sc. Carola Trahms, University of Kiel, Department of Computer Science, cat@informatik.uni-kiel.de
Supervisors:
- Prof. Dr. Martin Visbeck, GEOMAR Helmholtz Centre for Ocean Research Kiel and University of Kiel, Ocean Circulation and Climate Dynamics, mvisbeck@geomar.de
- Prof. Dr. Matthias Renz, University of Kiel, Department of Computer Science, mr@informatik.uni-kiel.de
Location: Kiel
Disciplines: computer science, statistics, oceanography
Keywords: knowledge bases, information retrieval, machine learning, data mining
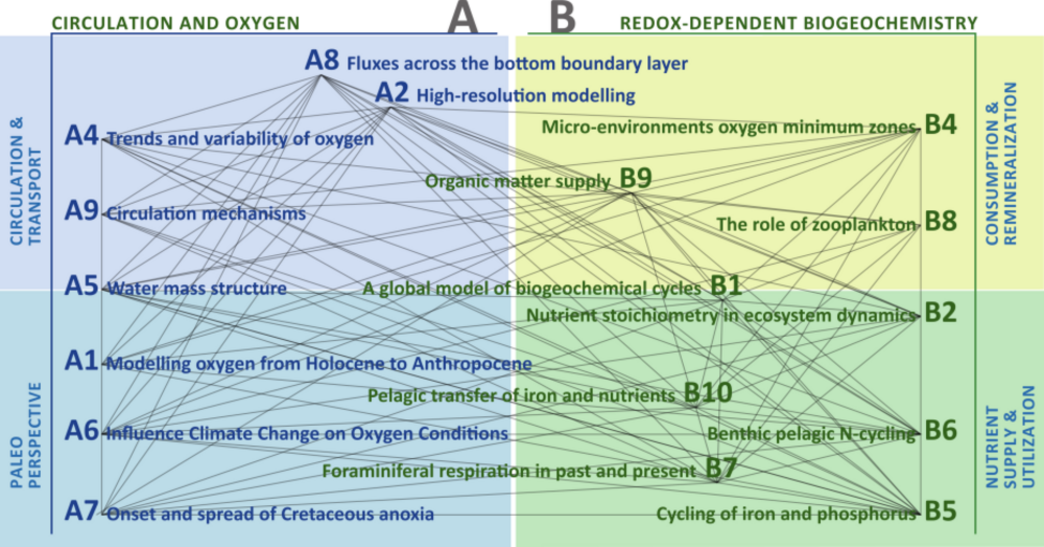
Objective: The availability of huge amounts of data, specifically marine science data, is rapidly increasing providing a valuable source of information for marine data driven science. However, data is still too often collected, managed and stored in an isolated project- or scientific question-specific way, usually used to address a specific question, yielding individual isolated data and knowledge silos. The main objective of this project is to unlock the tremendous power of knowledge that can be derived by fusing multiple disparate (but potentially connected) datasets across diverse domains of marine data. The semantical interconnected micro-level data and corresponding context information projected into one structure has huge potential to answer further advanced marine science questions. The challenge of this project is to identify and connect relevant information on micro level from datasets consisting of multiple modalities, each of which has a different representation, distribution, scale, and density. How to unlock the power of the extracted, potentially hidden, knowledge from these datasets is paramount in big data research, essentially distinguishing big data from traditional data mining tasks. This calls for advanced techniques that can fuse knowledge from various datasets organically in a machine learning and data mining task. Another challenge is the discovery of new insights based on novel information-network-structure based pattern mining approaches.
Aim: In this project, we want to investigate an interdisciplinary approach for building a central knowledge base by inter-connecting marine science data and context information by following the concept of cross-domain data fusion [Zheng 2015], a general applicable concept that has been introduced in the context of urban and transportation science. We aim to design novel algorithms to extract and exploit the knowledge derived from a highly heterogeneous and potentially unstructured source of marine data. Specifically, on the micro level, new approaches for information retrieval and pattern mining on complex-structured data including spatial and spatio-temporal information have to be developed. Furthermore, for the cross-domain data fusion task, machine learning methods for link prediction to discover hidden links between data need to be investigated. In addition, novel Heterogeneous Information Network (HIN) analysis concepts for discovering knowledge to answer domain questions and investigate hypothesizes on macro level need to be developed and investigated. As a use-case we will apply this concept on data from the Collaborative Research Center 754 "Climate-Biogeochemistry Interactions in the Tropical Ocean" (SFB 754). The SFB 754 is a 12 year long interdisciplinary research project studying the coupling of tropical climate and ocean circulation variability with the ocean's oxygen and nutrient balance. Since 2008 the SFB 754 has collected a broad range of observations from many marine disciplines. All data is freely available on the World Data Center Pangaea. It is quality controlled and enriched with meta-information to facilitate its reuse by other projects. Currently Pangaea hosts 690 SFB-related data sets (www.pangaea.de/?q=SFB754) with several hundred more to come in the near future. The SFB 754 has so far lead to 350 articles in peer-reviewed journals (oceanrep.geomar.de/cgi/search/quick?quick=sfb754). These articles form a second basis on which the proposed project can investigate the connectivity between the different data sets and disciplines. The broad range and free and easy availability makes this dataset an ideal test case to explore. Recent studies show that interconnected, multi-typed data represented as heterogeneous information networks (HINS) allow us to develop structural analysis approaches by leveraging the rich semantic meaning of structural types of objects and links in the networks [Patel et al., 2018, June; Patel et al., 2018, August]. In order to make best use of the heterogeneous dataset this project will require the adaptation and development of new algorithms for advanced machine learning and data mining. The incorporation of spatial, temporal and spatiotemporal attributes as well as the consideration of uncertainty of observations and findings in such networks have yet been little studied and will be a central element in this study. The overarching aim of this interdisciplinary project is to exemplarily prove the power of cross-domain data fusion as general concept useful to many other fields of marine science and beyond.
Tasks: 1) Exploiting datasets consisting of multiple modalities, each of which has a different representation, distribution, scale, and density; 2) Development of advanced machine learning and data mining techniques used to fuse the knowledge from various marine science datasets; 3) Identification of, potentially hidden, relationships between heterogeneous data: 4) efficient representation and organization of the data, metadata and relationships that serves as knowledge base (knowledge graph); 5) Advanced machine learning, data mining and information retrieval techniques to discover new and relevant information and infer knowledge from the knowledge graph; 6) the interpretation and incorporation of the newly discovered relationships in the framework of existing marine knowledge.
References:
- Zheng, Y. (2015). Methodologies for cross-domain data fusion: An overview. IEEE transactions on big data, 1(1), 16-34.
- Patel, H., Paraskevopoulos, P., & Renz, M. (2018, June). Data fusion of diverse data sources: enrich spatial data knowledge using HINs. In Proceedings of the Fifth International ACM SIGMOD Workshop on Managing and Mining Enriched Geo-Spatial Data (pp. 13-18). ACM.
- Patel, H., Paraskevopoulos, P., & Renz, M. (2018, August). GeoTeGra: A System for the Creation of Knowledge Graph Based on Social Network Data with Geographical and Temporal Information. In 2018 IEEE/ACM International Conference on Advances in Social Networks Analysis and Mining (ASONAM) (pp. 617-620). IEEE.